Andrei Rogobete: Artificial Intelligence & Management Theory
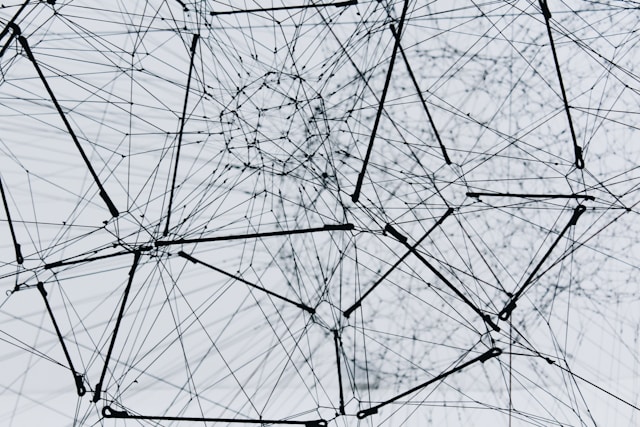
This paper is part of a series of essays that seek to explore the current and prospective impact of AI on business. A PDF version can be accessed here.
The advent of Artificial Intelligence (AI) upon the business world raises a myriad of challenges and opportunities for management theorists. The first of these is a matter of considered choice: Which are the most suitable theoretical lenses that one might apply in understanding the novel phenomena that AI represents? Might one start with Taylorism and the scientific management approach, or perhaps rather turn to Henri Fayol and his pioneering work on administrative management theory? Still, it may be wise to go back and consider Weber’s work on hierarchy and the resulting Bureaucracy Theory, or perhaps Elton Mayo’s advancements in Human Relations Theory and the creation of ‘humanistic organisations’.[1] Modern managerial thought (post-WW2) brought us the pioneering work of Joan Woodward and Contingency Theory which cannot be ignored. In the realm of psychology and the broader expansion of behavioural science and personnel management, we have Maslow’s influential Hierarchy of Needs and Douglas McGregor’s Theory X and Theory Y. Far from being exhaustive, this list illustrates the plethora of avenues that are available to the inquisitive researcher. This paper, however, elects what many might consider a less obvious choice – that is, an analysis of AI through Peter Drucker’s writings and more specifically, through his concept of the ‘Knowledge Worker’.
It is important to note from the onset that when referring to AI here we are referring specifically to Generative AI, which represents a branch of the wider field that is artificial intelligence, the main distinction being that generative AI has the capacity to learn and produce novel output autonomously.
In an article in the California Management Review during the winter of 1999, Drucker made a compelling statement:
The most important, and indeed the truly unique, contribution of management in the 20th century was the fifty-fold increase in the productivity of the manual worker in manufacturing. The most important contribution management needs to make in the 21st century is similarly to increase the productivity of knowledge work and knowledge workers. The most valuable assets of a 20th-century company was its production equipment. The most valuable asset of a 21st-century institution (whether business or non-business) will be its knowledge workers and their productivity.[2]
Throughout his lifetime Peter Drucker proved to be a prolific writer, having published some 41 books and countless articles, essays and lectures. The totality of his work amounts to over ten million words which as one scholar put it, is the equivalent of 12 Bibles or 11 Complete Works of Shakespeare.[3] No wonder then, that his alias as the ‘Father of Modern Management’ is a fitting title.
Drucker was born in Vienna in 1909 into a Lutheran protestant family.[4] His father was a lawyer and civil servant, and his mother studied medicine – both parents were considered intellectuals at the time. His house often served as a place of congregation for scientists, academics, and government officials, who would meet and discuss new ideas.[5] Yet his formative years were spent at Hamburg University, where he read international law and became heavily influenced by the works of Kierkegaard, Dostoevsky, Aquinas, Luther, Calvin and Weber. Here he developed a sense of Christian responsibility In tackling life’s challenges and made it his life mission to discover a society‘…in which its citizens could live in freedom and with a purpose’.[6] Interestingly, he was not swayed by Marxism because, in his view, the will of the collective came at the expense of the freedom and purpose of the individual: ‘there was no capacity for individual purpose in a collective society’, Drucker remarked.[7]
Amongst scholars and business executives he is perhaps best known for his work on decentralisation and a management approach that emphasises the value of employees and their contributions in achieving the shared goals of the organisation. His most celebrated theory is Management by Objectives (MBO Theory), initially presented in his 1954 book, The Practice of Management,[8] which was later refined in his 1974 magnum opus, Management: Tasks, Responsibilities, Practices.[9] Yet, Peter Drucker’s most distinguished and lasting contribution came in bringing about a novel way of understanding the field of management as an integrative whole. Previous writers such as Rathenau, Fayol, and Urwick drew connections between the varied functions of management, but it took Drucker to tie in all the strings and establish Management as a standalone discipline of study and practice.[10]
Drucker also fundamentally changed how employees were to be viewed by the company. He was the first to argue that they represent assets, not liabilities, and that within the modern economy, employee value and development is crucial to the well-being of the organisation.[11] Indeed, it is in the company’s best interest to invest and support career learning and the continual growth of its employees.
The Effectiveness of Knowledge Workers
Drucker first introduced the concept of a ‘knowledge worker’ in his 1967 book, The Effective Executive, where he defined it as ‘…the man who puts to work what he has between his ears rather than the brawn of his muscles or the skill of his hands’.[12]
He understood and foresaw the seismic shift that the well-developed economies of the West would experience in transitioning from a largely manual workforce to a predominantly knowledge-driven economy. The kickstart to all of this was, of course, the revolution in Information Technology (IT) from the 1950s onwards. Drucker explains that: ‘Today, however, the large knowledge organisation is the central reality. Modern society is a society of large organised institutions. In every one of them, including the armed forces, the centre of gravity has shifted to the knowledge worker’.[13]
What, then, makes the knowledge worker valuable? It is, put rather crudely, his or her ability to make a contribution to the firm. Unlike manual workers of the past, the knowledge worker benefits from a degree of heightened indispensability since the driving source of their effectiveness lies not in machinery or even in skill, but in the knowledge and judgement found between their ears. The concept of effectiveness becomes a key theme in Drucker’s writing on the knowledge worker: ‘…[those] schooled to use knowledge, theory and concept rather than physical force or manual skill work in an organisation and are effective only in so far as they can make a contribution to the organisation’.[14]
This raises questions surrounding the measurability of effectiveness of knowledge workers. It is important and interesting to note that throughout the 1950s the term ‘productivity’ was not yet in widespread use, hence Drucker’s reliance on ‘effectiveness’ as an early substitute. The traditional methods of measurement applied to manual work would no longer apply to knowledge work. The ‘yardsticks’ used for manual work such as industrial quality control or total output generation are ill-fitted to the knowledge worker. The knowledge worker also cannot be monitored ‘closely or in detail’, such an effort is futile for the organisation.[15] Instead, all efforts must be concentrated on the effectiveness of the knowledge worker. Drucker here usefully points out that unlike the manual worker, the knowledge worker produces immaterial things: knowledge, ideas, and concepts that remain unquantifiable in a physical sense. Instead, the ultimate task of the knowledge worker is to convert these abstract intangibles into tangible effectiveness for the organisation, this being in stark contrast to the manual worker who needn’t have to undergo this step of conversion – their contribution being already justified by the goods produced. Therefore, ‘Knowledge work is not defined by quantity. Neither is knowledge work defined by its costs. Knowledge work is defined by results’.[16]
If the knowledge worker ‘thinks’ in his or her contribution to the firm and this ‘thinking’ yields favourable results for the organisation, then surely the principal aim of the knowledge worker is to develop and grow their thought processes. In this sense they are all executives because they possess the capacity as well as the permission (given by their superiors or the company in general), to enact impactful decisions that are a direct result of their thinking.[17] Surely, then, the following challenge is one of discernment in differentiating the right decisions amidst the wrong ones. Within such a context, how might knowledge worker effectiveness be gained?
Drucker argues that it has nothing to do with personality traits: ‘Among effective executives I have known and worked with, there are extroverts and aloof, [others] even morbidly shy. Some are eccentrics, others painfully correct conformists. Some are worriers, some are relaxed. […] Some are men of great charm and warmth, some have no more personality than a frozen mackerel’.[18]
If personality traits have little to no bearing on effectiveness, or at least there is no evidence to prove the contrary, what does have an impact on effectiveness? Drucker argues that effectiveness ‘…is a habit, that is a complex of processes’. There is no silver bullet when it comes to seeking knowledge worker effectiveness, rather, it represents a collection of practices and habits that collectively amount to favourable results for the employee as well as the organization. The beauty of it is that practices and habits can be learned, meaning that any knowledge worker has the capacity to become effective.
However, as Drucker points out, ‘practices are simple, deceptively so; […] practices are always exceedingly hard to do well’.[19] There are five key practice areas that executives and knowledge workers need to master should they wish to become ‘effective’. The first is time – an effective knowledge worker knows what their time is mostly spent on and controls the allocated time that they have at work. The second is a focus on outward contribution – keeping one’s ‘eye on the ball’ so to speak. The effective knowledge worker always maintains an awareness of the overarching goal which helps direct the smaller practices and offers mental guideposts in achieving the desired outcomes. The third area is a sober awareness of one’s strengths and weaknesses. Effective workers build upon their strengths – be those inherent, personal strengths or the strengths conferred on them by their position within the organisation. The fourth area is the ability to distinguish what approaches are likely to yield the most impactful results and focus primarily on them. The fifth and final area is a fundamental understanding of the decision-making process and how to navigate it to make effective decisions. They are aware of operating within a system where too many, sometimes hasty, decisions, can lead to poor outcomes. Only a carefully thought-through strategy will result in favourable outcomes in the long-run.[20]
Drucker and Technology: The Impact of AI Upon the Knowledge Worker
What would the likely impact of AI be on the knowledge worker within such a context? The aforementioned five areas of practice offer multiple viewpoints for one to postulate how AI might augment (or replace), the daily activities of the knowledge worker.
When it comes to matters of automation and the arrival of new technologies, Peter Drucker warns against a position of extremes: technology is seldom a total panacea or an absolute disaster.[21] Indeed, in 1973 he pointed out that, ‘The technology impacts which the experts predict almost never occur’.[22] Drucker would have experienced the early hype surrounding digitalisation and the purported gifts of computing in the 50s and 60s. In some of his earlier writings he branded the computer a ‘mechanical moron’ – one that is very able at storing and processing precise data yet omits all that represents unquantifiable data, the problem of course being that it is often exactly this ‘unquantifiable data’ that becomes essential to the success of the organisation in the long-run.[23] It is often not the trends themselves that dictate a company’s future but rather changes in trends and the unique events which, at least in the early stages, are yet to be quantifiable. They are too nascent to become ‘facts’ and by the time they do become facts it is often too late. Drucker points out that the logical ability of computers represents both their biggest strength and their biggest weakness. One advantage that humans hold over the machine is their enhanced sense of perception and intuition. However, there is a serious risk that executives (i.e. all knowledge workers), might lose this sense of perception if they rely too heavily on quantifiable, computable data at the expense of unquantifiable, qualitative data.[24] This is a behavioural challenge that needs emphasising.
AI: Data versus Information
The resulting key theme that emerges in Drucker’s writing is the notion of data versus information. It’s relevance to analysing the potential consequences of AI lie within the wider scope of using software to effectively manage data. The crux of the problem is as follows: data, in its raw form, is inconsequential until it is interpreted and acted upon. Too many knowledge workers are ‘computer literate’ but not ‘information literate’: they know how to access data but aren’t adept at using it.[25]
For over half a century, Drucker argues, there has been an overwhelming focus on the ‘T’ in IT and the development of technology that stores, processes, transmits and receives data, but not enough effort has been placed on the ‘I’: What does this data mean to me? What does it mean to my business? What purpose does it serve? These are all fundamental questions that haven’t been given the prominence they deserve. [26] The main challenge is to ‘…convert data into usable information that is actually being used’.[27] This has ultimately resulted in decades of computer technology serving as a producer of data and not a producer of information. Drucker, quite rightly, points out that computer generated information has had practically no impact on a business deciding whether or not to build a new office, or a county council deciding to build a new hospital, a prison, a school and so on.[28] The computer has had minimal impact on high-level decisions in business.
Yet this is not just a failure of technology or even of some form of stubbornness amongst knowledge workers and executives; it is principally a failure of providing relevant information that is needed to perform and/or change the direction of any given task.[29] This effort is personalised and applies to each individual worker or executive. The focus then shifts from data gathering to data interpretation but, as mentioned, also to an astute discernment in organising and acting upon said data. The availability of data becomes second to the usability of data. Efforts move toward organising, interpreting, and acting upon reliable data.
As information is the principal resource of knowledge workers, Drucker suggests three broad organisational methodologies. We will briefly detail each in part and thereafter consider the potential implications of AI.
The first is called Key Event which looks at one or multiple important events that have a major contributing role towards the end performance of the knowledge worker. [30] This can be a single event or, as it is often the case, a series of key events that may direct certain outcomes. The event(s) in this case act as a ‘hinge’ upon which performance is dependent. Any executive or knowledge worker stands to benefit substantially in his or her career if they are able to identify, interpret and act upon such events.
The second methodological concept is based on modern Probability Theory and its resulting Total Quality Management (TQM).[31] This approach looks at a variety of possible outcomes that are expected to fit within a given range (i.e. withing the normal probability distribution), and singles out the outliers (those that do not meet the criteria). These exceptional events automatically move from being data (where no action is needed), to being information which necessitates immediate action.[32] This approach is useful when overseeing something like a large manufacturing process but can also be applied to the provision of services, for instance, a client going bankrupt, a deal falling through, a project yielding unexpectedly poor results, etc.
The third methodology for organising information is similar to the second and is based upon the Threshold Phenomenon and the field of perception psychology pioneered by the German physicist Gustav Fechner (1801-1887).[33] This holds that humans only perceive events to become a phenomenon once they cross a certain ‘threshold’ – and the threshold itself is subjective to each individual. In physical pain we only experience it once the stimuli are of such an intensity that they become categorised as ‘pain’. Similarly, it is the intensity and/or frequency of certain data points that lead to their recognition as phenomena. Drucker argues that accurately identifying the phenomena can assist knowledge workers (or managers, executives) in the early prediction of trends. The threshold concept is highly useful in identifying which sequences of events are likely to become trends and require immediate attention.
Conclusions: striving toward AI as a generator of useful information
How might AI assist within this context? The methodologies of organising data are effectively attempts to filter out and sieve the critical information from what otherwise is a plethora of largely useless noise. AI has a major role to play not merely in data monitoring and gathering but increasingly in extraction and accurate interpretation. Here lies the biggest challenge: which AI Large Language Model (LLM) will emerge as the most capable and useful to the knowledge executive?
The reality is that there are likely to be several dominant LLMs with differing traits and characteristics. It is becoming increasingly clear that a multimodal system will benefit from the advantage of being able to receive and work across differing types of data, including text, images, sound, and video. However, multimodality alone won’t suffice if the AI performs poorly at data interpretation and reasoning (e.g. hallucinations, general black box optimisation issues, and so on). We are currently in the nascent stages of a more in-depth, multi-layered reasoning approach with companies such as Meta and OpenAI investing heavily in the ability of AI chatbots to reason memorise, and comprehend more complex challenges. OpenAI’s chief operating officer Brad Lightcap said that in the near future, ‘We’re going to start to see AI that can take on more complex tasks in a more sophisticated way. […] I think we’re just starting to scratch the surface on the ability that these models have to reason. [Today’s AI systems] are really good at one-off small tasks, [but they are still] pretty narrow in their capabilities’.[34]
Again, for AI to have a significant impact on the decisions of knowledge workers they need to possess the capacity to provide a consistent supply of relevant, actionable information. We can already see the underpinnings of a technological infrastructure that may facilitate this: continued growth in the Internet of Things (IoT), the proliferation of AI hardware and artificial neural engines in a rising number of products and services, the consolidation of reliable datasets used to train LLMs, the fine-tuning of AI chatbots with specific characteristics and so on. This also creates a pool of moral and ethical challenges for executives: issues around data privacy, misinformation, bias, fraud, manipulation (e.g. impersonating people to promote products or services via ‘Deepfakes’), the recurring problem of AI hallucinations and so on. All of these issues require careful consideration. However, at this stage the importance of AI’s primary function as a provider of useful information cannot be understated. It may well represent the pivotal element in determining the success or failure of generative AI within business and beyond.
Andrei E. Rogobete is Associate Director at the Centre for Enterprise, Markets & Ethics. For more information about Andrei please click here.
Bibliography
[1] O’Connor, Ellen, Minding the Workers: The Meaning of `Human’ and `Human Relations’ in Elton Mayo. Organization, 6(2), 223-246. https://doi.org/10.1177/135050849962004
[2] Drucker, F. Peter, Knowledge-Worker Productivity: The Biggest Challenge, California Management Review, 41(2): 79-94
[3] Malcolm Warner, Morgen Witzel, The Oxford Handbook of Management Theorists, Oxford: Oxford University Press, 2013, p. 271
[4] Drucker, F. Peter, The Ecological Vision: Reflections on the Human condition, 2016, p. 425.
[5] Beatty, Jack. The World According to Peter Drucker, Wisconsin: Magna Publishing, 2016, pp. 5–7.
[6] Malcolm Warner, Morgen Witzel, The Oxford Handbook of Management Theorists, Oxford: Oxford University Press, 2013, p. 272
[7] Ibid.
[8] Drucker, F. Peter, The Practice of Management, New York: Harper & Row, 1954.
[9] Malcolm Warner, Morgen Witzel, The Oxford Handbook of Management Theorists, Oxford: Oxford University Press, 2013, p. 281
[10] Ibid. p. 291
[11] Drucker, F. Peter, Collins, J., Kotler, P., Kouzes, J., Rodin, J., Rangan, V. K., et al., The Five Most Important Questions You Will Ever Ask About your Organization, New Jersey: Wiley, 2008, p. xix
[12] Drucker, F. Peter, The Effective Executive, New York: Harper Collins, 1966, p. 3
[13] Ibid.
[14] Ibid
[15] Ibid. p. 4
[16] Ibid. p. 7
[17] Ibid. p. 8
[18] Ibid. p. 22
[19] Ibid. p. 23
[20] Ibid p. 24-25
[21] Wartzman, Rick, ‘What Peter Drucker Had to Say About Automation’, Harvard Business Review, November 2nd, 2015, https://hbr.org/2015/11/what-peter-drucker-had-to-say-about-automation
[22] Drucker, F. Peter, Management: Tasks, Responsibilities, Practices, Milton Park: Routledge, 1973, p. 267
[23] Drucker, F. Peter, The Effective Executive, New York: Harper Collins, 1966, p. 16
[24] Ibid. p. 18
[25] Drucker, F. Peter, Managing in Times of Great Change, Cambridge: Harvard Business Review Press, 1995, p. 109
[26] Drucker, F. Peter, Management Challenges in the 21st Century, Milton Park: Taylor and Francis, 1999, p. 97
[27] Drucker, F. Peter, Managing in Times of Great Change, Cambridge: Harvard Business Review Press, 1995, p. 113
[28] Drucker, F. Peter, Management Challenges in the 21st Century, Milton Park: Taylor and Francis, 1999, p. 99
[29] Ibid.
[30] Ibid. p. 127
[31] Ibid.
[32] Ibid.
[33] Ibid.
[34] Madhumita, Murgia, OpenAI and Meta ready new AI models capable of ‘reasoning’, The Financial Times, April 9th 2024, https://www.ft.com/content/78834fd4-c4d1-4bab-bc40-a64ad9d65e0d