Neil Jordan: (How) Does Artificial Intelligence Think? (What) Does it Know?
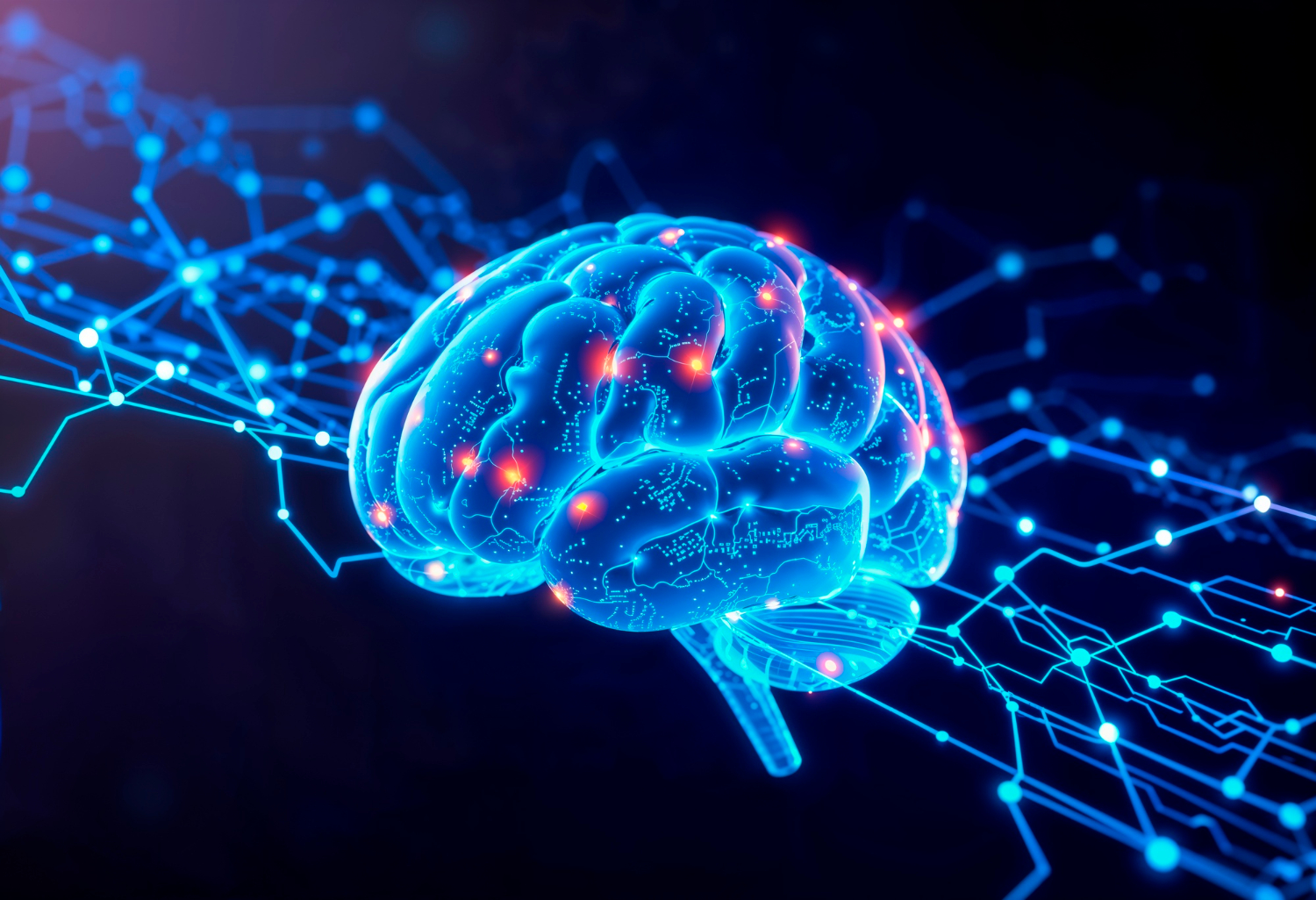
A recent article reports on work by researchers at Anthropic, the AI lab that developed a ‘reasoning’ AI model, and their ability to look into the digital brains of large language models. Investigating what happens in a neural network as an AI model ‘thinks’, they uncovered some unexpected complexity that would suggest that on some level, an LLM might have a grasp of broad concepts and does not simply engage in pattern matching. Conversely, there is evidence to suggest that when a reasoning AI explains how it has reached a conclusion, its account of how it has reasoned does not necessarily match what the ‘digital microscope’ suggests has gone on. Moreover, sometimes, an AI will simply produce random numbers in response to a mathematical problem that it can’t solve, and then move on. On occasion, it will respond to a leading question with reasoning that leads to the suggested conclusion, even if that conclusion is false. Thus, it seems, the AI will appear to convince itself (or the human interlocutor) that it has reasoned its way to a conclusion when in fact it has not.
The Human Foibles of AI
Should we consider this to be indicative of an approach towards human levels of intelligence or reasoning? After all, even the failings of AI are similar to our own. Most people have given up on a problem as being too difficult at some point in their lives, perhaps giving an inadequate account of their efforts to deal with it. Almost all of us, as school children, will have guessed at the answers to the questions in our maths books and shown some kind of working-out, even if we didn’t have any real confidence in what we had written. Similarly, if asked a difficult question in class, most of us will have attempted to give an answer that consisted of little more than repeating back to the teacher partial information – provided by the teacher in the first place and which we had not understood – in the hope of convincing him or her that we knew what we were talking about.
Perhaps, then, advanced AI models are closer to human beings than we have been willing to accept. If this is the case, where does the difference between AI reasoning and human reasoning lie? Or, rather, on what grounds can we say that human beings reason or think, while machines, however sophisticated, do not? What does it really mean to talk about computers ‘knowing’, ‘remembering’ or ‘working things out’?
Knowing That / Knowing How
There is a distinction to be drawn between conscious, propositional knowledge, and skill or aptitude – between ‘knowledge that’ and ‘knowledge how’. The former is a grasp of a matter of truth and can be held before the mind; the latter is something we are able to do and can often be executed with little thought. It is with this distinction in mind that we can easily agree with the philosopher and essayist Michel de Montaigne, who argued in his long essay An Apology for Raimond Sebond (1576), that if human beings can have knowledge, then animals surely can, too. When we look at the feats of which they are capable, beyond anything that human beings can manage without complex machinery and intricate calculations, how can we claim that they do not ‘know’? Surely a blackbird knows how to build a nest and a bumblebee knows when to hibernate? A spider’s web is a beautiful and intricate construction that any human being would struggle to build. Their levels of consciousness and their powers of reasoning are far below ours, yet in some sense they ‘know’ what they are doing (even if, we might claim that they do not know why or to what purpose – as a hamster probably does not have a long-term end in view when she hoards food and various other objects). If we think of the difference between the flight of a blackbird and what human beings have managed with aeroplanes and helicopters, the former is a natural aptitude, devoid of reason or thought, while the latter, cumbersome (yet ingenious) as it is, is based on vast array of theory, calculation and applied propositional knowledge.
Knowledge, Reason and Meaning
The distinction between knowledge how and knowledge that is not straightforward: there are various things we might know that might not cleanly fit into one category or the other – such as the knowledge that one is loved by one’s mother. There are also different ways of arriving at knowledge, some highly complex and based on extensive calculation, while others are simply intuitive. Similarly, there are different grades of reasoning, from basic connections of cause and effect or means and end, to highly abstruse relations between concepts. Nevertheless, when we ascribe to AI models the ability to reason or to know, we typically have in mind knowledge of the propositional variety: the AI model uncovers a fact or conclusion about some state of affairs, or provides an account of an expedient methods for achieving some goal, by way of a chain of steps.
Leaving aside the question of the extent to which animals possess such knowledge, we might say that in order to truly constitute knowledge, propositional knowledge requires the ability to understand. That is to say, for a human being to know, he or she must understand what is known, and where reasoning is involved, he or she must have a grasp of why the conclusion reached is valid. Moreover, he or she should understand what that piece of knowledge means in connection with other knowledge possessed, as part of a world filled with knowledge and meaning.
The Capacity of AI in the Absence of Consciousness
To illustrate this, we could point to the difference between solving a problem by understanding the nature of the problem itself, knowing the correct method for addressing it and understanding why the answer is right, and applying a process by conjecture, blindly following it and reaching a solution that might – or might not – be correct. Arguably the latter is still a form of reasoning, but a greatly diminished one. Even where the correct method is invariably applied and the solutions are always correct, the result surely does not constitute knowledge. In the absence of consciousness, these are the highest forms of reasoning and knowledge that AI can possess. When we say that a machine has reasoned or that it knows, we can really mean no more than this.
AI models can be incredibly efficient, solving problems and processing data more quickly than any human mind can manage; they can contribute to our knowledge and lead to hugely beneficial material improvements. For artificial intelligence, however, no matter how sophisticated and even when accompanied by an account of how a solution was reached, there is no knowledge or reasoning in the full, human sense. While a machine can disclose that the sun rose at 5:50 yesterday and can calculate the time of sunrise on any given date in the future, it cannot be said to know the time of sunrise. Between human beings and the most ingenious artificial intelligence, there remains the gulf of consciousness and the grasp of meaning that it renders possible. This might remain the case forever, but as machines approach more closely to human levels of intelligence, perhaps they will enable us to understand ourselves better and some of our own cognitive capacities.
Image: Designed by Freepik
Neil Jordan is Senior Editor at the Centre for Enterprise, Markets and Ethics. For more information about Neil please click here.